GPU, TPU, NPU Chips
NVIDIA's GPU - Graphic Chip Unit
The field of artificial intelligence (AI) is rapidly evolving, and the demand for AI-powered devices and applications is growing. This is driving the development of new AI chips, which are designed to accelerate AI workloads and improve performance while reducing the server footprint and cost..
There are a number of AI chip companies developing new chips, each with its own strengths and weaknesses include:
-
Nvidia: Nvidia is a leading graphics processing unit (GPU) manufacturer, and it has been a pioneer in the development of AI chips. Nvidia's AI chips are based on its GPU architecture, and they offer high performance and scalability. Nvidia's H100 is the most advanced AI chip available. In 2024, Nvidia is expected to release the Grace Hopper Superchip.
-
Graphcore: Graphcore is a British company that develops AI chips that are specifically designed for machine learning and artificial intelligence workloads. Graphcore's chips are based on its Intelligence Processing Unit (IPU) architecture, which is designed to accelerate neural network training and inference.
-
Cerebras Systems: Cerebras Systems is a California-based company that develops AI chips that are significantly larger than traditional chips. Cerebras' chips are based on its Wafer-Scale Engine (WSE) architecture, which allows for more processing power and memory bandwidth than traditional chips.
-
Intel: Intel is a leading semiconductor company that has been developing AI chips for several years. Intel's AI chips are based on its Xeon Scalable processor architecture, and they offer a range of features that are designed to accelerate AI workloads.
-
AMD: AMD is another leading semiconductor company that has been developing AI chips. AMD's AI chips are based on its EPYC processor architecture, and they offer a range of features that are designed to accelerate AI workloads.
-
Taiwan Semiconductor Manufacturing Company (TSMC) is the world's largest contract chipmaker, and it is based in Taiwan. TSMC produces chips for a wide range of companies, including Apple, Qualcomm, and Nvidia. TSMC is known for its high-quality chips and its ability to produce chips at a large scale. TSMC is a major player in the AI chip market. The company produces a range of AI chips, including the N5 and N4 chips. The N5 chip is based on the TSMC 5-nanometer process, and it offers up to 100 teraflops of performance. The N4 chip is based on the TSMC 4-nanometer process, and it offers up to 150 teraflops of performance. TSMC is investing heavily in AI chip development. The company is building a new fab in Arizona that will use the TSMC 5-nanometer process. The fab is expected to be operational in 2024.
The choice of AI chip will depend on the specific needs of the application. For example, Nvidia's chips are a good choice for high-performance AI workloads, while Graphcore's chips are a good choice for machine learning and artificial intelligence workloads that require high throughput. Cerebras Systems' chips are a good choice for applications that require a large amount of processing power and memory bandwidth. Intel and AMD's chips are a good choice for a range of AI workloads, and they offer a variety of features and performance levels.
Here is a more detailed comparison of the A100, H100, and other specific chips:
-
The A100 is Nvidia's flagship AI chip, and it was first released in 2020. It is based on the Nvidia Ampere architecture, and it offers up to 40 GB of HBM2e memory and 450 teraflops of FP32 performance. The A100 is designed for a wide range of AI workloads, including deep learning, natural language processing, and computer vision.
-
The H100 is Nvidia's latest AI chip, and it was released in 2022. It is based on the Nvidia Hopper architecture, and it offers up to 80 GB of HBM3 memory and 600 teraflops of FP32 performance. The H100 is designed for even more demanding AI workloads, such as training and deploying large language models.
-
The IPU-7 is Graphcore's latest AI chip, and it was released in 2022. It is based on the Graphcore Intelligence Processing Unit (IPU) architecture, and it offers up to 460 teraflops of performance. The IPU-7 is designed for a wide range of AI workloads, including deep learning, natural language processing, and computer vision.
-
The WSE 2.0 is Cerebras' latest AI chip, and it was released in 2022. It is a wafer-scale chip that measures 46,225 square millimeters, and it offers up to 1.5 exaflops of performance. The WSE 2.0 is designed for the most demanding AI workloads, such as training and deploying large language models.
-
Intel's Xeon Scalable processors offer a range of features that are designed to accelerate AI workloads, including Intel Deep Learning Boost, Intel Gaussian Neural Accelerator, and Intel VNNI. These features can improve the performance of AI workloads by up to 3x.
-
AMD's EPYC processors offer a range of features that are designed to accelerate AI workloads, including AMD Zen 4 architecture, AMD Infinity Fabric, and AMD CDNA 2 accelerators. These features can improve the performance of AI workloads by up to 2x.
The field of AI chip development is rapidly evolving, and new companies are entering the market all the time. This is leading to increased competition and innovation, which is benefiting the AI community. As the demand for AI-powered devices and applications continues to grow, the need for powerful and efficient AI chips will only increase.
Here is a table that compares the A100, H100, and TSMC's N5 and N4 chips:
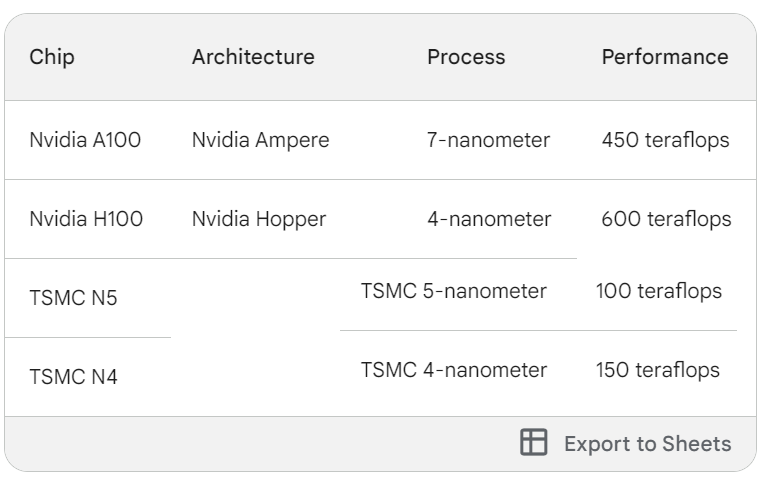
Google's TPU - Tensor Processing Unit
In the heart of Google's massive data centers lies a powerful engine driving innovation in artificial intelligence. This engine is the Tensor Processing Unit (TPU), a custom-designed chip that has revolutionized the way Google approaches AI workloads and continues to shape the future of the technology.
Unlike traditional CPUs and GPUs designed for general-purpose computing, TPUs are application-specific integrated circuits (ASICs), meaning they are specifically designed for the unique demands of machine learning and neural networks. This tailored architecture allows TPUs to achieve significantly higher performance and efficiency compared to conventional hardware.
Matrix Multiply Unit (MXU): This is the heart of the TPU, designed for the highly parallel computations required in machine learning algorithms. TPUs can perform matrix multiply operations with incredible speed and efficiency, exceeding conventional hardware by several orders of magnitude.
High-bandwidth memory: TPUs are equipped with high-speed memory interfaces optimized for the large datasets used in AI training and inference. This allows for seamless data flow and minimizes bottlenecks, further enhancing performance.
Scalability: TPUs can be easily scaled up to form large clusters, enabling them to tackle massive datasets and complex machine learning tasks. This scalability is crucial for Google's vast AI infrastructure and allows them to train and run large-scale models.
Energy efficiency: TPUs are designed to be energy-efficient, consuming significantly less power than traditional hardware while delivering superior performance. This is a crucial factor for Google, which operates massive data centers and must consider the environmental impact of its computing infrastructure.
TPUs have played a pivotal role in powering Google's various AI initiatives, including:
Search: TPUs are used to improve the accuracy and efficiency of Google Search, delivering more relevant and personalized results to users.
-
Translation: TPUs power Google's translation services, providing real-time translations across multiple languages with impressive accuracy.
-
Photos: TPUs enable Google Photos to automatically organize and enhance photos, detecting faces, objects, and events, and even suggesting creative edits.
-
Speech Recognition: TPUs improve the accuracy and responsiveness of Google Assistant and other speech-recognition systems.
-
YouTube Recommendations: TPUs personalize video recommendations on YouTube, suggesting content that users are more likely to enjoy.
-
Cloud AI Platform: Google's Cloud AI Platform provides access to TPUs and other AI tools for developers around the world, democratizing access to this powerful technology.
Google is continuously innovating and developing new versions of TPUs with even greater processing power and efficiency. The TPU v4 Pods, announced in 2022, offer a significant performance boost over previous generations, further solidifying TPUs as the backbone of Google's AI infrastructure.
Looking ahead, TPUs are expected to play an even more crucial role in the future of AI. As machine learning models become increasingly complex and data-intensive, TPUs will be essential for training and running these models efficiently. Additionally, the development of edge TPUs designed for mobile and embedded devices will further expand the reach of AI and unlock new possibilities for intelligent applications in our everyday lives.
In conclusion, Google's TPUs represent a remarkable achievement in hardware design and engineering. By specifically tailoring hardware for the unique demands of AI, TPUs have revolutionized the way Google develops and deploys intelligent technologies. As TPUs continue to evolve, they will undoubtedly play a major role in shaping the future of AI and driving innovation across various industries.
Neuromorphic Chips - Mimicking the Brain
The human brain remains one of the greatest unsolved mysteries. Its intricate network of neurons and synapses allows for unparalleled capabilities in learning, adaptation, and perception. Traditional computers, while powerful, have struggled to replicate these abilities due to their fundamentally different architecture. In recent years, however, a new paradigm has emerged: neuromorphic computing.
Neuromorphic chips, also known as neuromorphic processors or artificial neural networks (ANNs), are designed to mimic the structure and function of the brain. They utilize artificial neurons and synapses to process information in a parallel and distributed manner, similar to how the brain operates. This approach offers several potential advantages over conventional computing, including:
Low power consumption: The brain consumes significantly less energy than even the most efficient traditional computers. Neuromorphic chips aim to achieve similar energy efficiency by mimicking the brain's asynchronous and event-driven architecture.
High processing speed: The parallel nature of neuromorphic computing allows for faster processing of information, especially for tasks that involve pattern recognition and real-time decision making.
Adaptability and learning: Neuromorphic chips can be programmed to learn and adapt to new information, similar to how the brain forms new connections and pathways. This makes them ideal for applications such as machine learning and artificial intelligence.
Fault tolerance: The distributed nature of information processing in neuromorphic chips makes them less susceptible to errors and failures compared to traditional computers. This is because damage to individual neurons or synapses does not necessarily affect the overall functionality of the system.
The development of neuromorphic chips is still in its early stages, but it has the potential to revolutionize several fields, including:
Artificial intelligence: Neuromorphic chips could lead to the development of more powerful and efficient AI systems capable of performing tasks that are currently too challenging for traditional computers. This includes applications such as natural language processing, image recognition, and autonomous robotics.
Healthcare: Neuromorphic chips could be used to develop new diagnostic tools and treatments for neurological disorders. They could also be used to create more realistic and effective brain-computer interfaces for paralyzed patients.
Brain research: Neuromorphic chips could help scientists better understand the human brain by providing a physical model of its structure and function. This could lead to breakthroughs in our understanding of consciousness, learning, and memory.
Other applications: Neuromorphic chips could also be used in a variety of other applications, such as finance, cybersecurity, and self-driving cars.
Despite the promise of neuromorphic computing, there are still several challenges that need to be addressed before they can be widely adopted. These challenges include:
Hardware limitations: Current neuromorphic chips are still relatively expensive and energy-intensive compared to traditional computers. Additionally, they often lack the flexibility and programmability needed for certain applications.
Software development: Developing software for neuromorphic chips requires new tools and programming languages that are still in their infancy.
Algorithmic limitations: While neuromorphic chips excel at certain types of tasks, they are not yet a replacement for traditional computers for all applications.
Despite these challenges, the potential of neuromorphic computing is undeniable. As research and development continue, we can expect to see significant advancements in this field in the coming years. This will pave the way for a new era of computing that is more efficient, powerful, and adaptable than anything we have seen before.
Here are some additional points to consider:
Several companies are developing neuromorphic chips, including Intel, IBM, Samsung, and Qualcomm.
The European Union has launched a €1 billion initiative to accelerate the development of neuromorphic computing.
DARPA, the research arm of the US Department of Defense, is also investing heavily in neuromorphic computing research.
Leading Players:
-
Intel: Intel's Loihi chip is one of the most well-known neuromorphic processors, boasting a million artificial neurons and 256 million synapses. Intel's research efforts and open-source software tools like Lava contribute significantly to the field.
-
IBM: As the leading patent filer in neuromorphic computing, IBM actively pursues research and development through its TrueNorth chip and software ecosystem. Their focus on large-scale neuromorphic systems is notable.
-
BrainChip: This Australian company offers the Akida neuromorphic processor, known for its low power consumption and event-driven architecture. BrainChip has a strong focus on commercialization and partnerships, particularly in edge computing applications.
-
Qualcomm: This technology giant focuses on neuromorphic solutions for mobile devices, offering the Zeroth chip with its dedicated AI engine. Their expertise in mobile technology holds promise for on-device AI applications.
-
SynSense: This Swiss company focuses on neuromorphic vision processing with its DYNAPSE chip. Their technology offers high speed and efficiency for image recognition and analysis tasks.
With continued research and development, neuromorphic chips have the potential to transform our world and usher in a new era of computing. By mimicking the brain's remarkable capabilities, we can create machines that are more intelligent, efficient, and adaptable than ever before.
Most-Referred Neuromorphic Publications
-
"Deep Spiking Neural Networks: Models, Algorithms and Applications" by Yonghong Tian, Timothée Masquelier, Guoqi Li, Huihui Zhou, and Zhengyu Ma. Published in Frontiers in Neuroscience on September 15, 2023. (448 views)
-
"Sensory-inspired Event-Driven Neuromorphic Computing: creating a bridge between Artificial intelligence and Biological systems" by Alex James, Arindam Basu, Michel Paindavoine, and Guoqi Li. Published in Frontiers in Neuroscience on January 15, 2023. (6,379 views)
-
Most Advanced Neuromorphic Publications
-
"Training Multi-Layer Spiking Neural Networks with Plastic Synaptic Weights and Delays" by Jing Wang. Published in Frontiers in Neuroscience on December 4, 2023.
-
"Experimental demonstration of coupled differential oscillator networks for versatile applications" by Manuel Jiménez. Published in Frontiers in Neuroscience on November 30, 2023.
Please note that these publications are not necessarily the "best" in the field, but they have been cited and accessed frequently in recent months. This could be due to their novelty, impact on the field, or accessibility to a wider audience.
Here are some additional resources you may find helpful:
-
Neuromorphic Computing and Engineering: https://iopscience.iop.org/journal/2634-4386
-
Neuromorphic Engineering Section in Frontiers in Neuroscience: https://www.frontiersin.org/journals/neuroscience/sections/neuromorphic-engineering
-
Research Publications: TENNLab - Neuromorphic Architectures, Learning, Applications: https://neuromorphic.eecs.utk.edu/
-
Neuromorphic Computing Group: Publications: https://ncg.ucsc.edu/